Adaptive Radar and AI Shatters Performance
Using AI plus an open-source dataset could lead to rapid advancements in adaptive radar systems. Adaptive radar detects, locates, and tracks moving objects, rapidly taking pictures. With the help of modern AI approaches and lessons learned from computer vision, researchers at Duke University have broken through the performance barriers of adaptive radar.
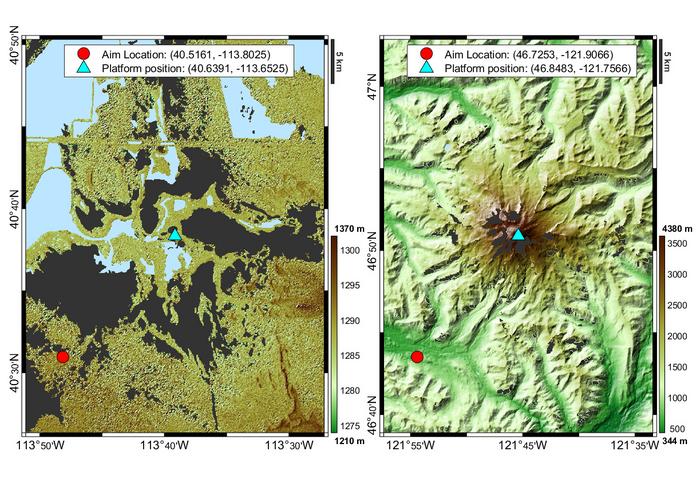
CREDIT: Duke University
In a paper published in the journal IET Radar, Sonar & Navigation, Duke engineers show that using convolutional neural networks (CNNs) can greatly enhance modern adaptive radar systems. They released a large dataset of digital landscapes for other AI researchers to build on their work.
While radar is not difficult to understand, as technology advances, so too have the concepts used by modern radar systems. It can shape and direct signals, process multiple contacts at once, and filter out background noise. However, radar has come as far as it can using these techniques alone—adaptive radar struggles to accurately localize and track moving objects, especially in complex environments.
In 2010, researchers at Stanford University released an enormous image database consisting of over 14 million annotated images called ImageNet. Researchers around the world used it to test and compare new AI approaches that became industry standard. The current collaborators show that using the same AI approaches greatly improves the performance of current adaptive radar systems.
So far, they benchmarked their AI’s performance on a modeling and simulation tool called RFView, which incorporates the Earth’s topography and terrain into its modeling toolbox. Then, they created 100 airborne radar scenarios based on landscapes across the contiguous United States and released it as an open-source asset called “RASPNet.” The researchers received special permission from the creators of RFView to build the dataset with more than 16 terabytes of data over several months and make it publicly available.
The scenarios included were handpicked by radar and machine learning experts and featured a wide range of geographical complexity. An example of a simple adaptive radar system scenario is the Bonneville Salt Flats, while the hardest is Mount Rainier. The team previously showed that an AI tailored to a specific geographical location could achieve up to a 7x improvement in localizing objects over classical methods. If an AI could select a scenario in which it had already been trained, it would substantially improve its performance.