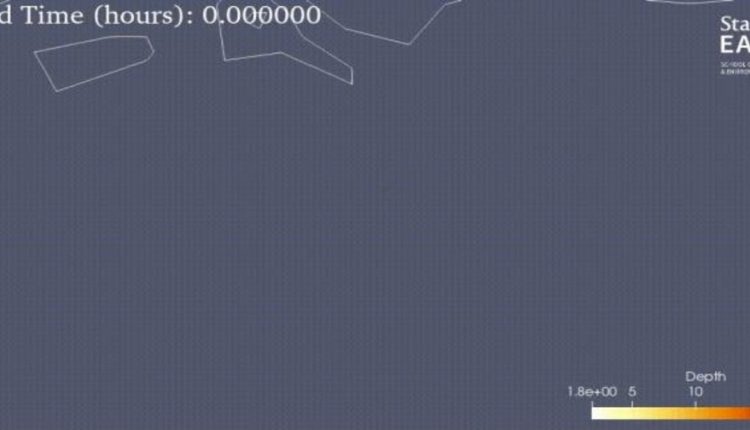
Earthquake Transformer Uses AI to Detect Small, Hidden Earthquakes
Small earthquakes (magnitude 2) occur several hundred times a day, with larger ones happening about once a year. But as more and more of the world is urbanized, even moderate earthquakes can cause devastation within cities.
As part of Mostafa Mousavi’s PhD studies in geophysics, he used to scan recorded earthquake signals, verifying that decades-old algorithms had detected true earthquakes rather than tremors generated by ordinary things like crashing waves, passing trucks or stomping football fans.
“I did all this tedious work for six months, looking at continuous data,” said Mousavi, now a research scientist at Stanford’s School of Earth, Energy & Environmental Sciences (Stanford Earth). “That was the point I thought, ‘There has to be a much better way to do this stuff.'”
This was in 2013. Handheld smartphones were already loaded with algorithms that could break down speech into sound waves and come up with the most likely words in those patterns. Using artificial intelligence, they could even learn from past recordings to become more accurate over time.
Seismic waves and sound waves aren’t so different. One moves through rock and fluid, the other through air. Yet while machine learning had transformed the way personal computers process and interact with voice and sound, the algorithms used to detect earthquakes in streams of seismic data have hardly changed since the 1980s.
That has left a lot of earthquakes undetected.
Big quakes are hard to miss, but they’re rare. Meanwhile, imperceptibly small quakes happen all the time. Occurring on the same faults as bigger earthquakes — and involving the same physics and the same mechanisms — these “microquakes” represent a cache of untapped information about how earthquakes evolve — but only if scientists can find them.
In a recent paper published in Nature Communications, Mousavi and co-authors describe a new method for using artificial intelligence to bring into focus millions of these subtle shifts of the Earth. “By improving our ability to detect and locate these very small earthquakes, we can get a clearer view of how earthquakes interact or spread out along the fault, how they get started, even how they stop,” said Stanford geophysicist Gregory Beroza, one of the paper’s authors.
Focusing on what matters
Mousavi began working on technology to automate earthquake detection soon after his stint examining daily seismograms in Memphis, but his models struggled to tune out the noise inherent to seismic data. A few years later, after joining Beroza’s lab at Stanford in 2017, he started to think about how to solve this problem using machine learning.
The group has produced a series of increasingly powerful detectors. A 2018 model called PhaseNet, developed by Beroza and graduate student Weiqiang Zhu, adapted algorithms from medical image processing to excel at phase-picking, which involves identifying the precise start of two different types of seismic waves. Another machine learning model, released in 2019 and dubbed CRED, was inspired by voice-trigger algorithms in virtual assistant systems and proved effective at detection. Both models learned the fundamental patterns of earthquake sequences from a relatively small set of seismograms recorded only in northern California.
In the Nature Communications paper, the authors report they’ve developed a new model to detect very small earthquakes with weak signals that current methods usually overlook, and to pick out the precise timing of the seismic phases using earthquake data from around the world. They call it Earthquake Transformer.
According to Mousavi, the model builds on PhaseNet and CRED, and “embeds those insights I got from the time I was doing all of this manually.” Specifically, Earthquake Transformer mimics the way human analysts look at the set of wiggles as a whole and then hone in on a small section of interest.
People do this intuitively in daily life — tuning out less important details to focus more intently on what matters. Computer scientists call it an “attention mechanism” and frequently use it to improve text translations. But it’s new to the field of automated earthquake detection, Mousavi said. “I envision that this new generation of detectors and phase-pickers will be the norm for earthquake monitoring within the next year or two,” he said.
The technology could allow analysts to focus on extracting insights from a more complete catalog of earthquakes, freeing up their time to think more about what the pattern of earthquakes means, said Beroza.
Hidden faults
Understanding patterns in the accumulation of small tremors over decades or centuries could be key to minimizing surprises — and damage — when a larger quake strikes.
The 1989 Loma Prieta quake ranks as one of the most destructive earthquake disasters in U.S. history, and as one of the largest to hit northern California in the past century. It’s a distinction that speaks less to extraordinary power in the case of Loma Prieta than to gaps in earthquake preparedness, hazard mapping and building codes — and to the extreme rarity of large earthquakes.
Only about one in five of the approximately 500,000 earthquakes detected globally by seismic sensors every year produce shaking strong enough for people to notice. In a typical year, perhaps 100 quakes will cause damage.
In the late 1980s, computers were already at work analyzing digitally recorded seismic data, and they determined the occurrence and location of earthquakes like Loma Prieta within minutes. Limitations in both the computers and the waveform data, however, left many small earthquakes undetected and many larger earthquakes only partially measured.
After the harsh lesson of Loma Prieta, many California communities have come to rely on maps showing fault zones and the areas where quakes are likely to do the most damage. Fleshing out the record of past earthquakes with Earthquake Transformer and other tools could make those maps more accurate and help to reveal faults that might otherwise come to light only in the wake of destruction from a larger quake, as happened with Loma Prieta in 1989, and with the magnitude-6.7 Northridge earthquake in Los Angeles five years later.
“The more information we can get on the deep, three-dimensional fault structure through improved monitoring of small earthquakes, the better we can anticipate earthquakes that lurk in the future,” Beroza said.
Earthquake Transformer
To determine an earthquake’s location and magnitude, existing algorithms and human experts alike look for the arrival time of two types of waves. The first set, known as primary or P waves, advance quickly — pushing, pulling and compressing the ground like a Slinky as they move through it. Next come shear or S waves, which travel more slowly but can be more destructive as they move the Earth side to side or up and down.
To test Earthquake Transformer, the team wanted to see how it worked with earthquakes not included in training data that are used to teach the algorithms what a true earthquake and its seismic phases look like. The training data included one million hand-labeled seismograms recorded mostly over the past two decades where earthquakes happen globally, excluding Japan. For the test, they selected five weeks of continuous data recorded in the region of Japan shaken 20 years ago by the magnitude-6.6 Tottori earthquake and its aftershocks.
The model detected and located 21,092 events — more than two and a half times the number of earthquakes picked out by hand, using data from only 18 of the 57 stations that Japanese scientists originally used to study the sequence. Earthquake Transformer proved particularly effective for the tiny earthquakes that are harder for humans to pick out and being recorded in overwhelming numbers as seismic sensors multiply.
“Previously, people had designed algorithms to say, find the P wave. That’s a relatively simple problem,” explained co-author William Ellsworth, a research professor in geophysics at Stanford. Pinpointing the start of the S wave is more difficult, he said, because it emerges from the erratic last gasps of the fast-moving P waves. Other algorithms have been able to produce extremely detailed earthquake catalogs, including huge numbers of small earthquakes missed by analysts — but their pattern-matching algorithms work only in the region supplying the training data.
With Earthquake Transformer running on a simple computer, analysis that would ordinarily take months of expert labor was completed within 20 minutes. That speed is made possible by algorithms that search for the existence of an earthquake and the timing of the seismic phases in tandem, using information gleaned from each search to narrow down the solution for the others.
“Earthquake Transformer gets many more earthquakes than other methods, whether it’s people sitting and trying to analyze things by looking at the waveforms, or older computer methods,” Ellsworth said. “We’re getting a much deeper look at the earthquake process, and we’re doing it more efficiently and accurately.”
The researchers trained and tested Earthquake Transformer on historic data, but the technology is ready to flag tiny earthquakes almost as soon as they happen. According to Beroza, “Earthquake monitoring using machine learning in near real-time is coming very soon.”
Source: Stanford University