MIT’s new computer algorithm could enhance photos, make them more memorable
You’ve probably heard of subliminal images, which the brain picks up sometimes without a human even being aware. But how well do you actually remember images you see throughout the day?
Researchers from MIT’s Computer Science and Artificial Intelligence Laboratory (CSAIL) have developed an algorithm that can predict how memorable (or forgettable) an image is, almost to the human level. The researchers plan on using it to create software that can make minor changes to photos and make them more memorable— a technique that can come to benefit the advertising industry, as well as help develop better teaching resources.
MIT’s “MemNet” algorithm creates a heat map that points out which parts of an image are most memorable. If the team was to enhance the different regions, it could potentially increase how well a person remembered the image.
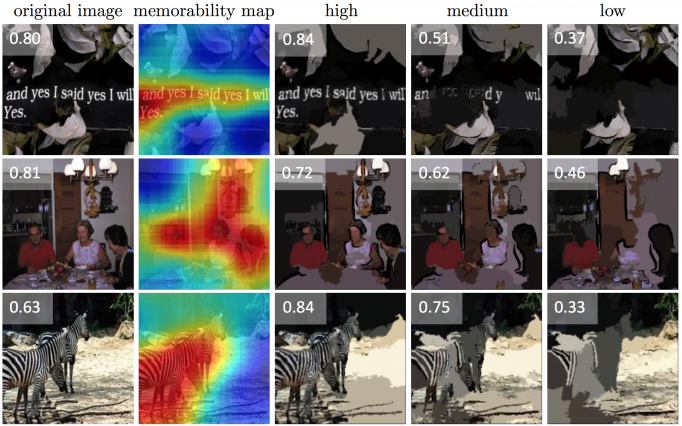
“Understanding memorability can help us make systems to capture the most important information, or, conversely, to store information that humans will most likely forget,” said Aditya Khosla, CSAIL graduate student and lead author on a related paper. “It’s like having an instant focus group that tells you how likely it is that someone will remember a visual message.”
As part of the study, the research team also published the world’s largest image-memorability dataset, LaMem, which includes 60,000 images that are marked up with metadata about qualities like popularity and emotional impact. The team developed LaMem in an attempt to spark further research in computer vision.
So how does the algorithm work?
Taking what it learned from a previous study on facial memorability , the team created the system with techniques from the artificial intelligence field called “deep-learning,” which uses “neural networks” to teach computers to sort through data and come up with patterns, with no human interference. (The deep-learning technique is applied to Apple’s Siri, Google’s auto-complete, and Facebook’s photo-tagging.) The more data the network receives, the more it will adjust to form more accurate predictions.
The team used the algorithm on tens of thousands of images from datasets. The images weer given a “memorability score” that was based on how well humans remembered them.
After coming up with a score, the researchers tested the algorithm against humans, asking the model to predict how memorable humans would find a particular image. What they found was that it performed 30% better than any algorithms that were previously developed and was even close to human performance.
Going forward, the team will update the system and try to get it to predict the memory of a specific person, so it can gear different images toward different individuals’ memory.
Comments are closed, but trackbacks and pingbacks are open.